Covid-19 represents a challenge for all of humanity.
Such a pandemic necessitates a multi-faceted and multi-disciplinary approach in trying to understand not only the pathology of the disease and its characteristics, but its molecular biology, the means of infection, and incubation periods.
The biological factors (of virus and host) aside, environmental and social factors play extensive roles in facilitating contagion and survival of the virus.
The urgency with which extensive data sets should be analyzed is clear, to prevent the spread of the virus and its ability to cause death and destabilization of society.
In the situation we find ourselves, data science together with machine learning algorithms and multi-agent simulations , are tools which scientists and governments may use to facilitate and accelerate understanding to enable effective decision making.
In the Covid19 pandemic, there are multiple variables that are interacting on a worldwide scale. This interaction creates emergent and to some extent unpredictable phenomena which makes their observation and study very difficult. Multi-agent simulation is a way to approach complex systems like Pandemics by simulating a subset of their characteristics, components and interactions. This allows the observation of properties that otherwise would not be readily inferred by looking at separate parts of the system, making it possible to develop experiments that help to understand and eventually predict the emerging dynamics.
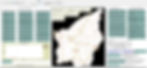
Application
The model presented here is a multi-agent simulation that visualises emerging dynamics from the interaction and influence of a small subset of multiple biological and social factors in the development of the covid-19 pandemic.
Given the scale of the pandemic, and the complexity of global societal structures, the simulation may not be viewed as a precise model of universal application. The simulation may however be tailored to locales to enable governments to assess strategic interventions and outcomes at local, municipal and regional levels. In facilitating such analysis, variables that play a critical role in the development of the pandemic have been singled out for manipulation in the model.
The variables so enabled, broken down by base line variable, are as follows:
- Population
o Initial population per age group
o Chance of infection per age group
o Chance of death per age group
o Number of people commuting per day
o Percentage of infection in commuters
- Clinical parameters
o Medical care capacity
o Initial infected population
o Average infection length
o Average time until symptoms show
o Average days for contagion to be possible
o Maximum distance for contagion to be possible
o Chance of having a severe infection
o Multipliying factor for chance of death in case of a severe infection
- Social interaction (being the number of places where groups of people of various age groups meet frequently)
o Number of schools
o Number of colleges
o Number of hospitality venues
o Number of adult venues
o Number of senior venues
o Number of public transport lines
o Number of food shops
Reporting
The model allows for experimentation with the above variables, and in so doing so allows users to observe the evolution of the infection and impact on the population over time.
It specifically visualizes and reports upon (overall and by age group) the number of:
- Healthy people
- Infected people
- Untreated cases of infected people
- Infected people receiving medical care
- Recovered people
- Deceased people
Strategizing intervention
In enabling scientists and governments to strategize responses and outcomes, the simulation incorporates a so ‘lock down’ switch. That is, to observe the development of the infection when the population is restricted in terms of mobility and interaction in public places.
The simulation has the possibility of being built out to incorporate additional variables, and strategic responses.
Actions and Orders
Setup: reset all variables and set the initial conditions for the simulation.
Go: runs the simulation. It can be paused/stop at any time by pressing the “go” button.
Complete lockdown: Per default is Off. When set to On it tells the simulation to send everyone home, stop all social interactions including use of public transport, stop commuters entering/leaving town and restricts movement of people.
Keep social distancing: tell people to keep a minimum set distance in metres from each other. The order is not followed accurately but more randomly and proportionate to the set distance. This variable is only taken into account when complete lock down is set to Off.
Prioritise elderly: Per default is Off. When set to On it tells the simulation to prioritise elderly patient (older > 60) access to hospital beds.
How to use it:
1. Scroll right or down as required to access the rest of the user interface.
2. Press the setup button.
3. Adjust the sliders of parameters according to the desired values.
4. Press the go button to start the simulation.
5. Observe plots at the bottom of the user interface. Plots can be disabled and enabled at anytime. Disabling plots can improve the speed of the simulation.
6. If there is need to change parameters or execute actions during the simulation, it is recommended to pause the execution using the go button. Change the parameters or execute orders as needed. Press go to continue with execution.
How it works:
Every tick of the simulation represents one hour.
The virtual world area shows a map outline in white (we chose the Republic of San Marino) with main roads outlined in yellow.
Non infected people are represented with green dots on the map.
Infected people are represented with red human shapes.
As the simulation runs, clusters of green dots will appear and dissipate. These correspond to the social gatherings of simulated people from different age groups in different types of venues and activities during the day. People using public transport gather mainly along the yellow lines in the map.
At the top of the map there are monitors indicating the following variables:
Days: Number of elapsed days since the simulation started.
% People infected: Percentage of people with ongoing infection.
# People infected: Number of people with ongoing infection.
# Untreated: Number of people with infection but not receiving medical care.
# People recovered: Number of people recovered from infection since the simulation started.
Medical care used: Number of hospital beds used.
Number of deaths: Number of people deceased since the simulation started.
Plots:
There are 3 plots at the bottom-left side of the screen. This plots show:
Population health: This plot shows the curves of:
- Healthy population
- Population infected with Covid19
- Population recovered from infection with Covid 19
Population infection with Covid19 per age group:
- 0-9 years
- 10-19 years
- 20-29 years
- 30-39 years
- 40-49 years
- 50-59 years
- 60-69 years
- 70-79 years
- >= 80 years
Deaths caused by infection with Covid19 per age group:
- 0-9 years
- 10-19 years
- 20-29 years
- 30-39 years
- 40-49 years
- 50-59 years
- 60-69 years
- 70-79 years
- >= 80 years
Click on the image below to download model: